Interaction Exists in a Multiple Regression Model When
Some sources say that the estimated model of a complete second degree polynomial regression model in two variables x 1 x 2 may be expressed as. Bmulticollinearity is present in a regression model.
Solved Q1 Interaction Exists In A Multiple Regression Model Chegg Com
I cant find a clear explanation of when an interaction term is necessary.
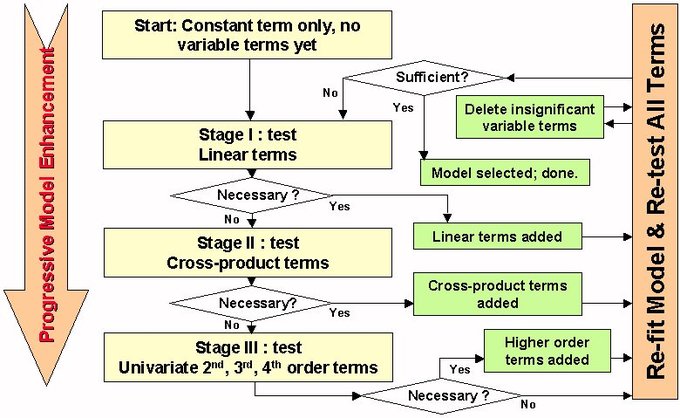
. If two independent variables are too highly correlated r2 06 then only one of them should be used in the regression model. An interaction occurs if the effect of an explanatory variable on the response variable changes according to the value of a second explanatory variable. Studied in Multiple Regression Analysis where x3 x1 x2.
If the degree of correlation between variables is high enough it can cause problems when you fit the model and interpret the results. We could get the same four simple effects tests from the full regression model. A phenomenon in which two or more predictor variables in a multiple regression model are highly correlated so that the coefficient estimates may change erratically in response to small changes in the model or data.
It is easy to understand how transformations work in the simple linear. Interaction exists in a multiple regression model when. In Continuous Predictors enter Temperature Pressure Time.
Y b 0 b 1 x 1 b 2 x 2 b 3 x 1 2 b 4 x 2 2 b 5 x 1 x 2. In this case the coefficients for the interaction are actually simple effects. An interaction occurs when an independent variable has a different effect on the outcome depending on the values of another independent variable.
We say that 2 variables interact when one influences the effect of the other. In figure 3 we have the OLS regressions results. Xk for each.
It shows that there is a significant malefemale difference for grp 1. Click Add next to Interactions through order 2. And others dont consider interaction term x 1 x 2.
The model to use in this case is. This is equivalent to a usual multiple regression model. We will be using statsmodels for that.
According to this model if we increase Temp by 1 degree C then Impurity increases by an average of around 08 regardless of the values of Catalyst Conc and Reaction Time. The logistic regression equation looks like below -. This chapter describes how to compute multiple linear regression with interaction effects.
Multicollinearity occurs when independent variables in a regression model are correlated. In multiple linear regression it is possible that some of the independent variables are actually correlated with one another so it is important to check these before developing the regression model. Interaction Effect in Multiple Regression.
The relationship between X1 and Y changes for differing values of X2. One independent variable affects the relationship between another independent variable and the dependent variable. When does an interaction occur in multiple regression.
A model without interactions assumes that the effect of each predictor on the outcome is independent of other predictors in the model. We transform the response y values only. The first step is to have a better understanding of the relationships so we will try our standard approach and fit a multiple linear regression to this dataset.
For example the first interaction coefficient is the simple effect of female at grp equal to one. Da polynomial model used. Adding interaction indicates that the effect of Tenure on the attrition is different at different values of the last year rating variable.
In Responses enter Strength. Theory guides the specification of appropriate interaction models using multiple regression analysis. To introduce basic ideas behind data transformations we first consider a simple linear regression model in which.
Select both Temperature and Pressure. Sometimes the dependent variable depends not just on the independent variables but also on the interaction between the variables. Cthe regression model is overall insignificant.
Multiple Linear Regression with Interactions. Cthe regression model is overall insignificant. Experimental unit in the sample.
Multicollinearity occurs when two or more independent variables are highly correlated with one another in a regression model. The presence of an interaction indicates that the effect of one predictor variable on the response variable is different at different values of the other predictor variable. That fits the regression model.
We transform both the predictor x values and response y values. This involves choosing which independent variables to include in. Neither one of 2 independent variables contribute significantly to the regression model.
Hypothesize the form of the model ie the deterministic component E y. In this case their main effectsthe separate effect of each of them on the outcome should no longer b. Click OK in all dialog boxes.
There is a curvilinear relationship between the dependent and independent variables. Logit p Intercept B1 Tenure B2 Rating Adding Interaction of Tenure and Rating. Adding a term to the model in which the two predictor variables are multiplied.
Traditional product terms with continuous variables assess interaction of a specific form namely bilinear interactions. We transform the predictor x values only. This correlation is a problem because independent variables should be independent.
An interaction term in a multiple regression model may be used when Select one. Navigate to Stat Regression Regression Fit Regression Model. Earlier we fit a linear model for the Impurity data with only three continuous predictors.
We postulate that the amount of votes a candidate gets. Use CTRL to multiselect. The principal finding is that interaction analysis is most straightforward when it is theoretically motivated.
By Jim Frost 188 Comments. This is equivalent to a usual multiple regression model. Collect the sample data ie the values of y x1 x2.
This is what wed call an additive model. Previously we have described how to build a multiple linear regression model Chapter ref linear-regression for predicting a continuous outcome variable y based on multiple predictor variables x. Two-Way Interactions Regression Models With Product Terms Continuous Predictors.
Solved Question Three Multiple Choice Choose Appropriate Chegg Com
How To Interpret P Values And Coefficients In Regression Analysis Statistics By Jim
Multicollinearity In Regression Analysis Problems Detection And Solutions Statistics By Jim
No comments for "Interaction Exists in a Multiple Regression Model When"
Post a Comment